Deep Learning, Medical Imaging
For prostate cancer patients, large organ deformations occurring between radiotherapy treatment sessions create uncertainty about the dose delivered to the tumor and surrounding healthy organs. Segmenting those regions on cone beam CT (CBCT) scans acquired on treatment day would reduce such uncertainties. In our latest work, a 3D U-net deep-learning architecture was trained to segment bladder, rectum and prostate on CBCT scans. Due to the scarcity of contoured CBCT scans, the training set was augmented with CT scans already contoured in the current clinical workflow. Our network was then tested on CBCT scans. The segmentation accuracy (as measured by the Dice similarity coefficient) increased significantly with the number of CBCT and CT scans in the training set, reaching a performance 10% better than conventional approaches based on deformable image registration between planning CT and treatment CBCT scans. Interestingly, adding CT scans to the CBCT training set allowed maintaining high performance, while halving the number of CBCT scans. Hence, our work showed that although CBCT scans included artifacts, cross-domain augmentation of the training set was effective and could rely on large datasets available for planning CT scans.
Collaborations
CHU-UCL-Namur, Belgium
CHU-Charleroi Hôpital André Vésale, Belgium
Links
Publisher: https://www.mdpi.com/2076-3417/10/3/1154
Github: https://github.com/eliottbrion/pelvis_segmentation
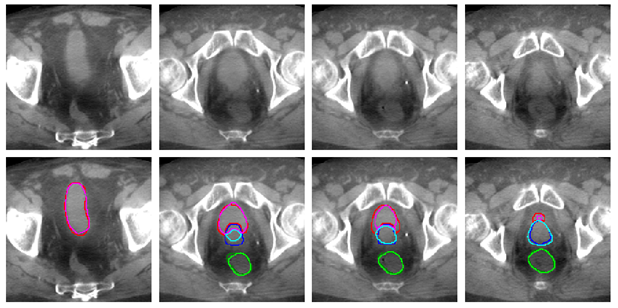
In this use case, the prostate is irradiated while minimizing the dose delivered to the healthy bladder and rectum. The image shows an example of automated bladder, rectum and prostate tracking on the CBCT scan acquired before each treatment cession. On this image, each column corresponds to a slice of the same CBCT scan. Dark colors represent the segmentation drawn manually by a human expert, while light colors show our algorithm segmentation. The automated bladder, in pink, rectum, in light green and prostate, in light blue, are close to human expert delineations. A further step will be to highlight these imporvements by showing better tumor coverage and reduction in the doses delivered to the healthy organs that it allows.